Digital markets are prone to “tipping.” Policymakers are starting to look at tipping as a market failure worthy of consideration. But as much as tipping is easy to understand, it is not simple to measure. An accurate and practical test for tipping is needed. One way is to focus on the behavior of the firm that tips the market.
A concept of “tipping” is gaining traction in the ever-changing policy landscape of the digital economy. At the close of 2020, the European Commission released its Digital Markets Act, an ambitious regulatory proposal in which it singles out markets with tipping potential as candidates for intervention.
In economics, tipping is the snowball effect that kicks in once a product crosses a critical point of user adoption, catapulting the supplier away from competition and towards a monopoly equilibrium. Markets that are prone to tipping are those with increasing returns to adoption on the demand side, also known as network effects. The rapid success of Google Search is a case in point.
The growing interest toward tipping by policymakers is welcome. To start, the economic literature has long recognized that tipping might lead to the selection of inferior technologies, and durable lock-in of consumers in inefficient outcomes. More important, perhaps, is that question marks continue to be placed on the ability of existing methods of monopoly power diagnosis to draw a fine line between competition and monopoly. In a recent complaint against Facebook, the US Federal Trade Commission’s application of traditional methods led it to disregard TikTok as a competitor to Facebook. This omission is remarkable, given that TikTok is the fastest-growing platform in the world. Recall also that Facebook did not bid when the former US administration tried to force the sale of TikTok to a domestic buyer. Facebook feared antitrust scrutiny on grounds of merger to monopoly issues. Perhaps, an inquiry into whether user groups distinct from Tiktok’s had tipped to Facebook would have provided stronger backing to the FTC’s monopoly charges.
“In economics, tipping is the snowball effect that kicks in once a product crosses a critical point of user adoption, catapulting the supplier away from competition and towards a monopoly equilibrium.”
This short piece suggests steps towards the formulation of a simple way to test for tipping. We write “simple” because unless one can think of a practical test that improves prediction without increasing application costs, the case for leaving traditional methods is weak. We start by shining a light on a largely-overlooked definition of tipping that focuses on the behavior of the firm that tips the market. This definition then allows for the design of a concrete test and a corresponding data strategy to determine whether tipping points have been crossed. Before we do this, however, we recall the economic case for testing for tipping.
Why (Ever) Measure Tipping?
Why should we care about tipping? A focus on tipping alleviates some of the difficulties involved with diagnosing market power in the digital economy. Conventional methods of evaluating market power rely on price and output metrics. In digital markets, this approach is hard to apply, because users are often “bartering,” rather than “paying.” They barter their personal data for otherwise free access to digital services. Google, for instance, offers various services at a zero monetary price, ranging from Google Search and Maps, to Translate and Docs. And when prices do exist, the complex structures found in multi-sided markets create analytical problems. Output measures are no less problematic. In the digital sector, it is hard to determine what the relevant measure of output is. Take search engines. Multiple indicators of output compete: search queries, page visits, user numbers, click-through, advertisement impression, and advertisement conversion. The variety of data points for output confronts antitrust analysts with considerable selection problems.
The comparative advantage of an empirical approach focused on tipping lies in its potential to improve this state of affairs. Rather than asking whether a firm has power over price or controls a monopoly share of output, the intellectual inquiry consists in asking whether a firm has crossed the “tipping point.” This is not an easy question, but the economic definitions provide useful directions.
Towards a Better Definition of Tipping
The conventional definition of tipping focuses on a firm pulling away from its competitors once it gains an initial advantage. The market tips when a winner takes most—if not all—of the market. When tipping is seen through these lenses, the obvious corollary for antitrust analysts is to look for monopoly as evidence of tipping. This leaves us back where we started.
What is often lost is that tipping has a behavioral implication. Supply is “lazy.” Around the tipping point, a snowball effect kicks in: The winner records user growth, without having to expend much effort. This effect, in turn, arises because demand is “sticky.” The winning firm can raise price or maintain a sluggish attitude to innovation, yet benefit from what Jean Tirole called “lucky demand conditions” in relation to utilities. The firm that gets ahead continues to get increasingly ahead, without needing to worry about demand echoing John Hicks’s classic statement that “the best of all monopoly profits is a quiet life.”
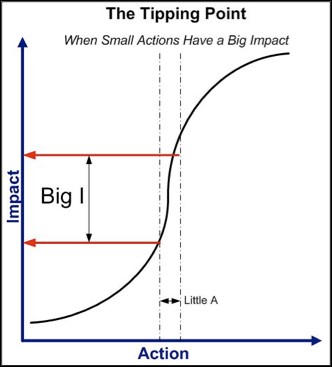
A Concrete Tipping Test
With eyes away from monopoly shares and towards inertial behavior, we can move closer to a concrete test of tipping. What is the firm under inquiry actually doing to expand demand? If the answer is “not much,” the market has likely crossed the tipping point. If the answer is the firm hustles to grow demand, the market might not yet have crossed the tipping point. The question then consists of identifying data points suggestive of firm effort.
Prices are an obvious starting point. It could be symptomatic of tipping that demand keeps increasing with prices. For example, AOL’s subscriber numbers kept growing in 1998 when it raised subscription prices in 1998. Similarly, firms that phase out freemiums or free trials might just be reaping the benefits of tipping. For example, Google Photos will start limiting its hitherto unlimited free storage to 15 GB later this year. In 2016, Adobe reduced the free trial period for its Creative Cloud lineup of apps, which includes Photoshop, from 30 days to 7 days. But these examples should not obscure the fact that price points and trends are not easy to observe in digital markets. Moreover, it is well accepted that firms should be allowed a certain degree of leeway to capture value from past investments with price increases. An undue focus on price hikes would render a tipping test excessively rigorous, biased towards findings of tipped markets.
More helpful insights into the degree of effort can be found on the firm’s expenditure side. Specifically, we can look at the expenses it incurs to grow demand in the short term. Marketing and advertisement expenses spring to mind. Firms that have crossed the tipping point need not invest in growth hacking, customer acquisition, or content creation. For example, Spotify has been ramping up its podcast offerings by signing exclusive million-dollar deals with buzzy names such as Joe Rogan, Prince Harry and Meghan Markle, Barack Obama and Bruce Springsteen, and Kim Kardashian. Apple has signaled an intention to make similar deals. Investments in content creation with an eye to attracting users suggest that the podcast distribution market remains, thus far, below the tipping point.
What does this mean, concretely? A table produced in the context of a Competition and Markets Authority market study in the UK in 2020 gives an illustration. The CMA sought to reconstruct the revenue and costs of Google Search as a standalone business, as opposed to the entire Alphabet Group. The costs that are demand expanding—and hence relevant to an inquiry into tipping—are those labeled direct costs or “Traffic Acquisition Costs.” By contrast, the category “other direct costs” contains essentially variable costs that increase as the demand for Google Search expands. They are irrelevant in the context of a tipping inquiry.
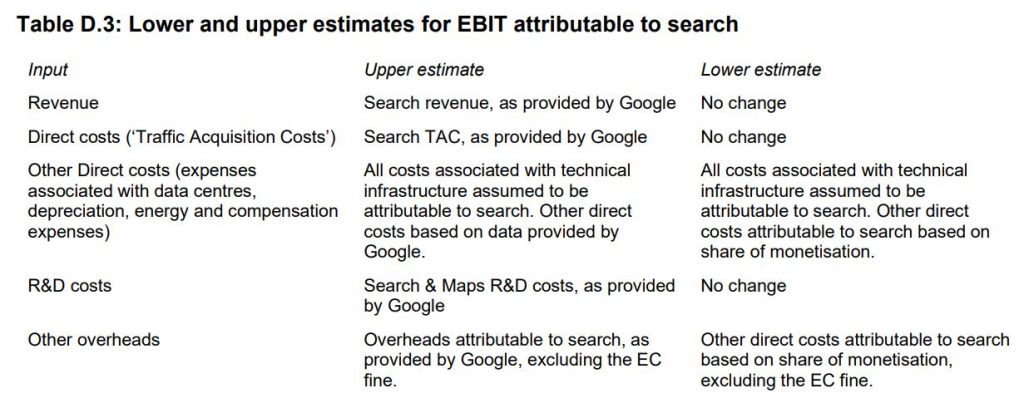
It is hard, though, to put exact numbers on the levels of expenditure below which we can speak of a tipped market. But in a way, this does not matter. What matters is this: if individual firm level data shows a clear discontinuity in pricing, marketing advertisement or content creation expenditure in the persistent presence of user growth, there is a telling sign that a tipping point has been crossed.
A Possible Data Strategy
A key challenge is that tech firms’ financial reporting often does not break down expenditure at the product, service, or application level. The large marketing expenditure reported by Apple every year, for example, says little about how and where Apple spends these dollars. If we want to assess the effort Apple puts into in the App Store, we need data specific to the App Store. A way forward for antitrust decision makers is to require disaggregated data of firms under investigations.
There is every reason to believe that tech firms have disaggregated data at their disposal. A quick search in the job openings that tech companies post on LinkedIn is illustrative. We learn for example that Apple runs an App Store “Team,” and other smaller related “teams” (like the “App Store editorial design team,” the “App Store Business Operations team,” and the “App Store Engineering R&D team”), with their own employees, and also likely budget and resources.
A starting point for a decision maker would thus be to ask large digital firms for organizational data, and focus the investigation on the resources spent in the segments, divisions, units and teams with direct involvement into the product, application or service under inquiry. This, in turn, would allow a finer grained understanding of a firm’s degree of effort over markets suspected of tipping. Besides, the obtained data might provide useful qualitative tidbits. For example, if the App Store team’s hiring expenditure is primarily aimed at hiring app reviewers, rather than marketing experts and content developers, this could indicate that Apple is more in demand maintenance mode than in demand expansion mode.
Conclusion
The most fatal objection to our approach concerns the costs of information. In his Nobel Prize lecture, Tirole stressed the importance of “information light” policies—policies that do not rely on information to which regulators are unlikely to have access to. Besides the fact that firms probably own more disaggregated data than we suspect, we want to stress here that the structural analysis which dominates antitrust is all but information light.
The market power evaluation requires market level, and sometimes industry level data, from all actual and potential suppliers of substitutes. Moreover, direct demand estimation techniques create exacting methodological and informational issues. Our tipping test targets one firm only. It does not require market data from other companies. Thus, an approach focused on tipping can lessen the costs of monopoly diagnosis.