Income inequality may exacerbate the spread of infectious diseases. In a new paper, Jay Bhattacharya, Joydeep Bhattacharya, and Min Kyong Kim examine the relationship between income inequality and the incidence and prevalence of tuberculosis across countries.
Rising income inequality has been blamed for many societal ills—crime, soak-the-rich politics, social anxiety, violence, lack of social cohesion—the list goes on and on. Researchers have noted an association between income inequities and various health measures: life expectancy, stress, heart disease, depression, and others. While it seems reasonable to expect that most of these health metrics correlate with poverty, it is not readily apparent why they should be associated with income inequality. Motivated to understand the devastation caused by the COVID-19 pandemic, we ask, is income inequality an added contributor to the spread of infectious disease? That is, are income inequality and the spread of infectious disease related beyond the association with income levels?
How might income inequality and infectious disease spread be connected? Consider two areas—or neighborhoods, to be more concrete—X and Y. Both areas are poor, with similarly low per capita incomes, but with income inequality substantially higher in X than in Y. Further, consider two inhabitants of area X: individual A, who is impoverished, and B, who is rich. It is reasonable to expect B to be healthier than A because B is richer. It also seems logical to suppose that people like A are significantly more prone to catching, harboring, and transmitting airborne infectious diseases, such as tuberculosis (TB). Finally, suppose people like A and B randomly mix and linger in the social settings of area X, such as schools, churches, workplaces, and public transportation. We posit infections are more likely to spread or linger in area X than in Y. We label this the “mixing hypothesis.”
Ideally, we would like to test the hypothesis directly. This method involves recording, in advance, the infectivity status and incomes of all individuals in a sample, meticulously observing who they interact with and for how long, and noting changes in their infectivity over a specific period. Using this approach would be the only way to identify a causal mechanism connecting inequality with disease spread. Such data, however, are unavailable and, some may argue, impossible to collect.
Leaving that sort of endeavor to future research, we simply look for a robust association between income inequality and the spread of infectious disease by conducting an ecological study using publicly available panel data for a large cross-section of countries between 1995 and 2013. Ecological studies are a form of observational research that examines data at the population or group level, rather than at the individual level. These studies are often used to evaluate the prevalence and incidence of diseases, particularly when the diseases are rare, by analyzing data in populations or groups instead of individual subjects.
The data include TB incidence (new cases) and prevalence (stock of existing cases), and income inequality (Gini coefficient) across countries and across time within countries. Figure 1a plots the logarithm of TB incidence, defined as new cases per 100,000 people in a year, versus the logarithm of the Gini coefficient of income for 97 countries in 2010. Figure 1b plots the logarithm of TB incidence versus the logarithm of per capita income across the same countries and year.
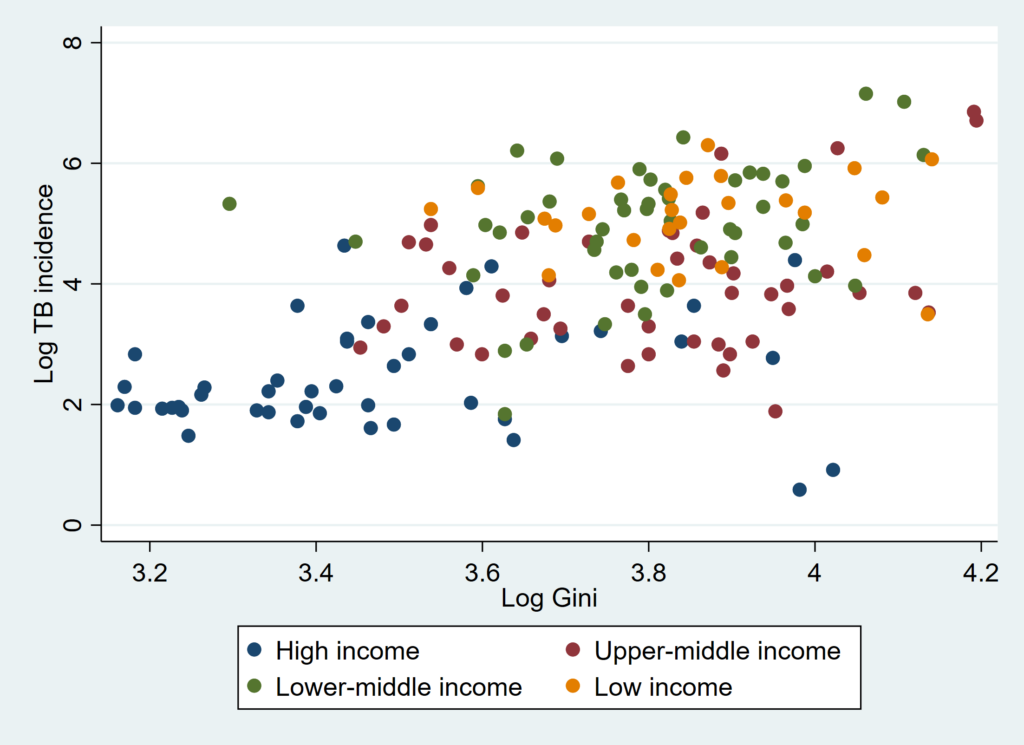
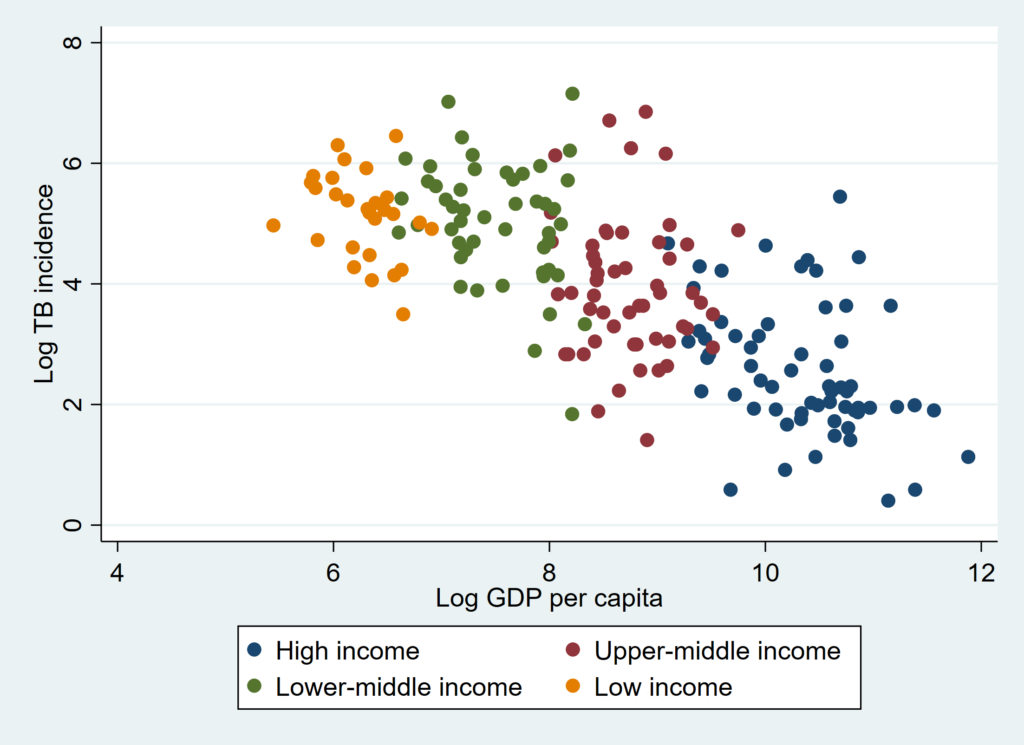
A quick look at the figures indicates a clear, positive association in Figure 1a and a negative association in Figure 1b. Delving deeper, our multivariate regression model controlled for, among other variables, economic output per capita, HIV prevalence, public health expenditures, population density, age composition, and poverty, while incorporating country-level and time-fixed effects.
Overall, elevated levels of income inequality were positively associated with tuberculosis prevalence. All else the same, countries with Gini coefficients of income a mere 10% apart see a statistically significant 4% difference in tuberculosis incidence.
Some threats to our methodology are easily identified. First, in countries with high income inequality, there is a significant population of poor individuals who are more susceptible to infections, while the number of wealthy individuals is relatively small. That, in and of itself, can explain why two countries with similar per capita incomes but different levels of income inequality can show different levels of TB prevalence. Second, the well-known “aggregation bias,” which emerges due to the non-linear relationship between income and health at the individual level, creeps in whenever analysts test hypotheses about individual-level relationships with data that averages individual-level data. Another channel, distinct from social mixing, such as low political support for public health, could drive the association.
These threats, in concert, raise the obvious question: Why should anyone believe in the robustness of the association we discover? This is where our use of a “negative control”—anemia—is vital. Anemia, like tuberculosis, is a disease prevalent in impoverished populations and thus needs to be addressed through the aforementioned challenges, such as relatively larger poor populations in countries with high income inequality, as well as government support for public health initiatives. Furthermore, the likelihood of developing anemia is also influenced by income in a non-linear manner, which suggests that the problem of aggregation bias may also be present. Therefore, all of the potential threats that come with our methodology are applicable to anemia as well, with the only difference being that it is a non-communicable disease. We find no association between anemia prevalence and income inequality in our data. This finding offers indirect credence to the mixing hypothesis.
Ultimately, our work is best seen as hypothesis-generating, rather than hypothesis-testing. Future research analyzing contact tracing data may help. The nature of the disease, and its pathogenesis, are also crucial. Our results rely on TB data and may not extend to other infectious diseases like HIV.
If our mixing hypothesis is confirmed by future work using income and social contact data, it will assert that income redistribution could be an essential policy lever to improve population health.
Articles represent the opinions of their writers, not necessarily those of the University of Chicago, the Booth School of Business, or its faculty.